Mainak Pal | মৈনাক পাল
c@pnamk..ii80gmalaomal
I am currently pursuing a Ph.D. in Electrical & Computer Engineering with a specialization in Automatic Control at Purdue University, where my research is supervised by Prof. Vijay Gupta. My work focuses on the development of algorithms to enhance teaming between humans and autonomous systems in uncertain environments. My research interests also include operator learning, bayesian modeling for multi-agent reinforcement learning.
I completed my Bachelor of Engineering in Electronics and Telecommunication Engineering from Jadavpur University, Kolkata, India. During my undergraduate, I had worked with Dr. Amit Konar on visual cognition. I have interned at Serre Lab, Brown University where I worked on behavioral analysis of worm locomotion, under Dr. Thomas Serre. Additionally, I worked on Zero-shot Learning at the VIP Lab, IIT Bombay, guided by Dr. Biplab Banerjee, and explored Natural Language Processing at the NLP Lab, Jadavpur University, where Dr. Sudip Kumar Naskar advised me. After graduation, I worked as a Data Scientist at Tata Steel, leveraging reinforcement learning for process optimization.
CV  / 
Google Scholar  / 
LinkedIn  / 
Github
|
|
Updates
- [Jan 2024] paper on Resilient MARL submitted to IEEE Transactions on Automatic Control.
- [Aug 2022] Started my PhD in Electrical and Computer Engineering at Purdue University. I will be working with Prof. Vijay Gupta.
- [Aug 2021] Joined Tata Steel as Data Scientist. I will be working with Analytics and Insights Team.
- [Jun 2021] Completed my B.E. with 1st Class Honors.
- [Mar 2021] paper on Zero-shot breast cancer segmentation is now available online.
- [May 2020] our work has been accepted for publication as a book chapter at Interpretable Artificial Intelligence - A Perspective of Granular Computing, Springer-Verlag.
- [May 2020] preprint of our work on transductive Zero-shot Learning is now available.
- [Apr 2020] I'll be interning at Serre Lab, Brown University over Summer 2020.
- [Aug 2019] awarded the RUSA 2.0 Travel Grant to present at ICCCMLA-2019, Goa, India
- [Jul 2019] paper on vector quantization clustering accepted at ICCCMLA-2019.
- [Mar 2019] I'll be interning at VIP Lab, IIT Bombay over Summer 2019.
- [Jan 2019] paper on sentiment analysis accepted at SemEval-2019.
Show More...
|
Research Experience
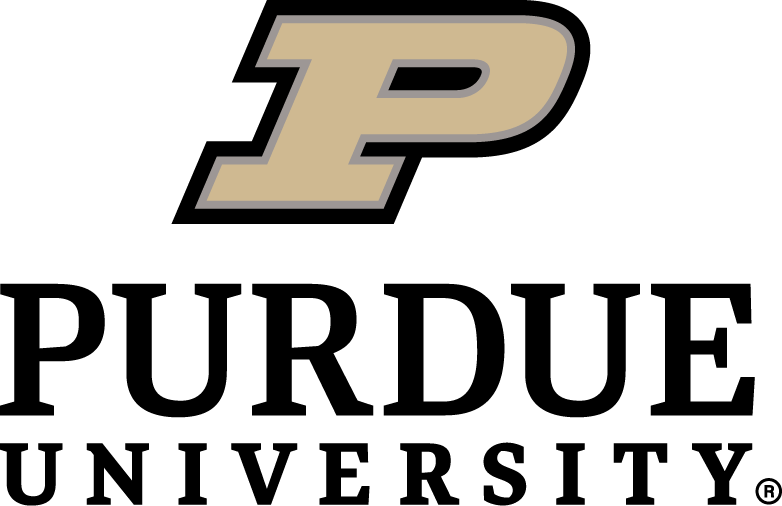 |
PhD Student, Graduate Research AssistantAug. 2022 - Present
Electrical and Computer Engineering, Purdue University, USA
Advisor: Dr. Vijay Gupta
|
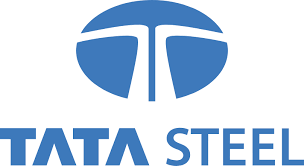 |
Data ScientistAug. 2021 - Aug. 2022
Analytics and Insights Team, Tata Steel, Kolkata, India
Chief: Amit Chatterjee
|
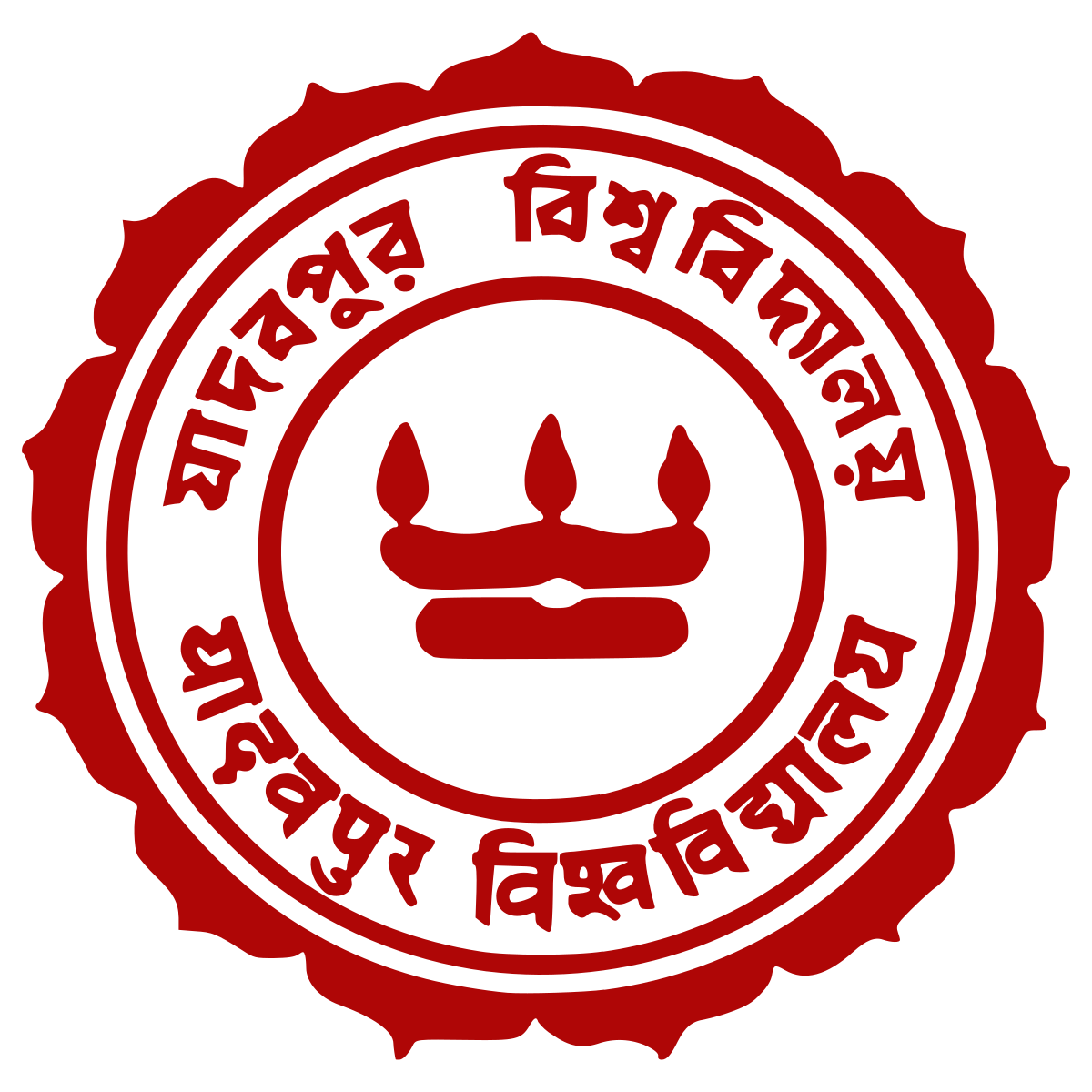 |
Undergraduate Research AssistantApr. 2019 - Jun. 2021
Artificial Intelligence Laboratory, Jadavpur University, India
Advisor: Dr. Amit Konar
|
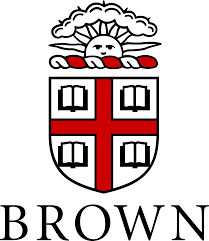 |
Summer Research InternMay. 2020 - Sep. 2020
Serre Lab, Brown University, USA
Advisor: Dr. Thomas Serre
|
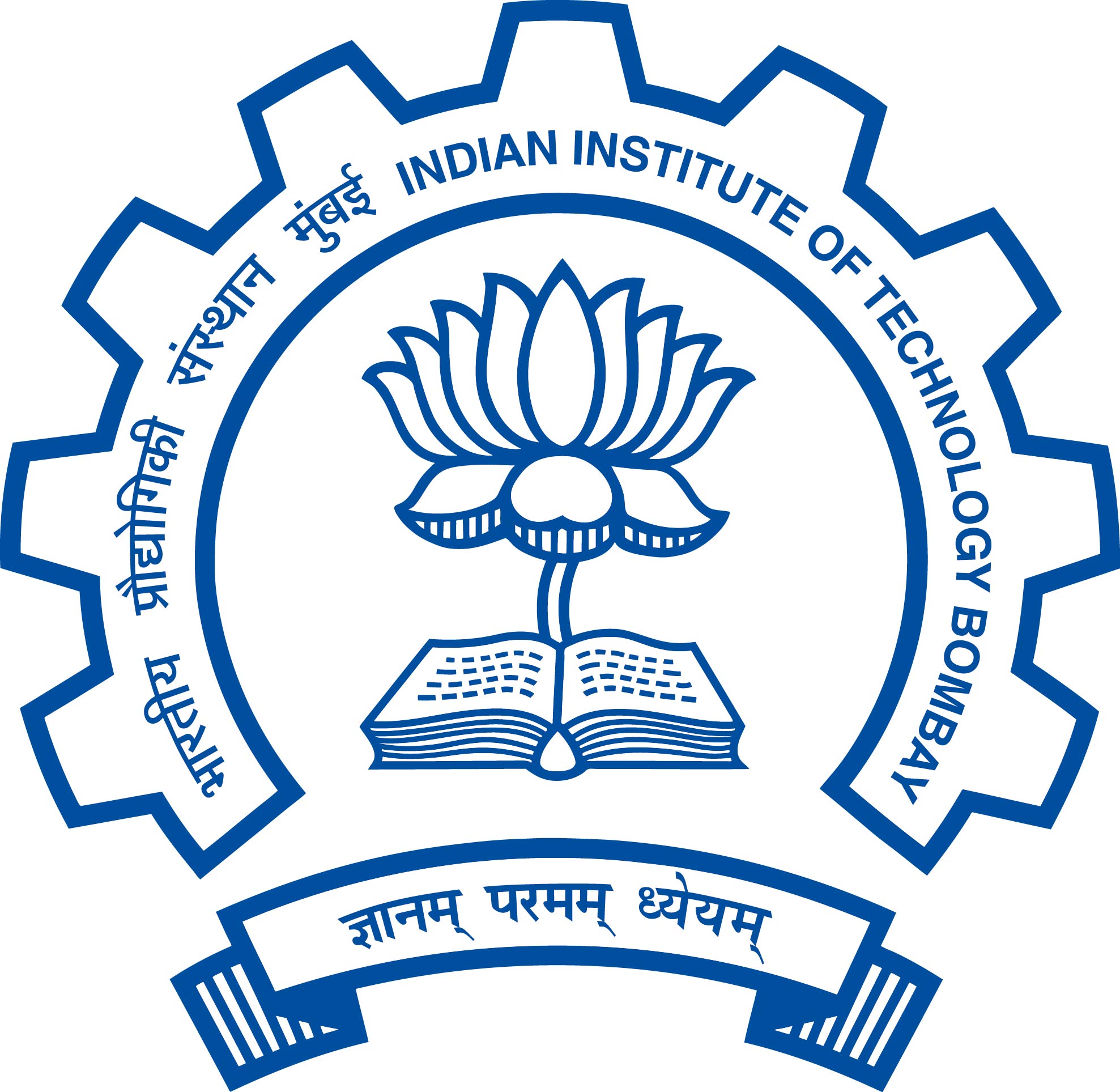 |
Summer Research AssistantMay. 2019 - Jul. 2019
VIP Lab, IIT Bombay, India
Advisor: Dr. Biplab Banerjee
|
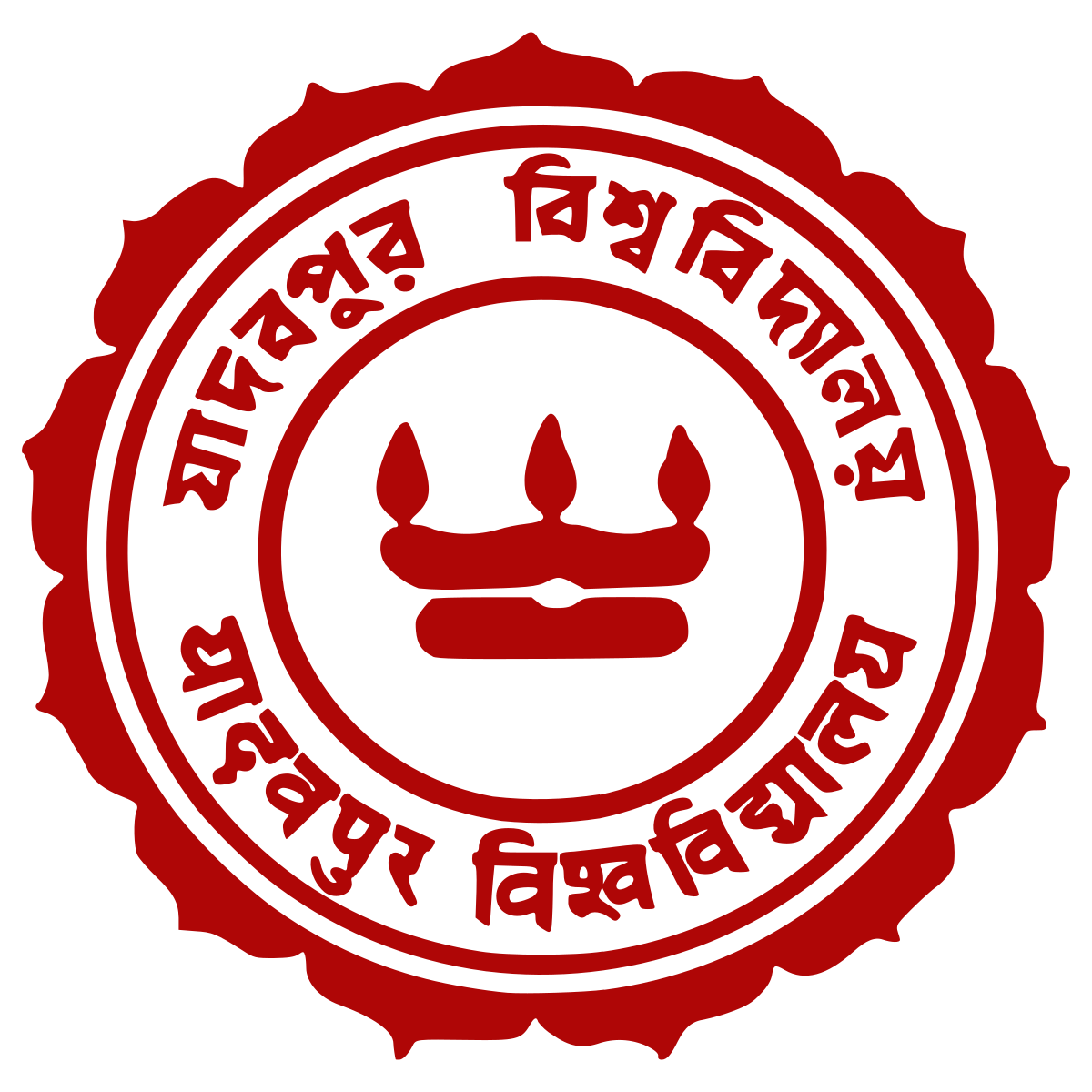 |
Undergraduate Research AssistantAug. 2018 - May. 2019
NLP Lab, Jadavpur University, India
Advisor: Dr. Sudip Kumar Naskar
|
Publication
*indicates equal contribution
|
|
Resilient Multi-agent Reinforcement Learning with Function Approximation
Lintao Ye, Martin Figura, Yixuan Lin, Mainak Pal, Pranoy Das, Ji Liu, and Vijay Gupta
Under review at IEEE Transactions on Automatic Control
project /
abstract /
code
Adversarial attacks during training can strongly influence the performance of multi-agent reinforcement learning algorithms. It is, thus, highly desirable to augment existing algorithms such that the impact of adversarial attacks on cooperative networks is at least bounded. We consider a fully decentralized network, where each agent receives a local re- ward and observes the global state and action. We propose a resilient consensus-based actor-critic algorithm, whereby each agent estimates the team-average reward and value function, and communicates the associated parameter vectors to its immediate neighbors. We show that in the presence of Byzantine agents, whose estimation and communication strategies are completely arbitrary, the estimates of the cooperative agents converge to a bounded consensus value with probability one, provided that there are at most H Byzantine agents in the network that is (2H + 1)-robust. Furthermore, we prove that the policy of the cooperative agents converges with probability one to a bounded neighborhood around a stationary point of their team-average objective function under the assumption that the policies of the adversarial agents asymptotically become stationary.
|
|
A Generative Model Based Approach for Zero-shot Breast Cancer Segmentation Explaining Pixels’ Contribution to the Model’s Prediction
Preeti Mukherjee*
Mainak Pal*,
Lidia Ghosh,
Amit Konar
Interpretable Artificial Intelligence - A Perspective of Granular Computing, Springer-Verlag
project /
paper /
abstract /
bibtex
Deep learning has extensively helped us to analyze complicated distributions of data and extract meaningful information from the same. But the fundamental problem still remains—what if there is no or rare occurrence of any instance which needs to be predicted? This is a significant problem in the health-care sector. The primary motivation of the work is to detect the region of anomaly in the context of breast cancer detection. The novelty here lies in designing a zero-shot learning induced Generative Adversarial Network (GAN) based architecture which has the efficacy to detect anomaly and thereby to discriminate the healthy and anomalous images even if the network is trained with the healthy instances only. In addition, this work has also been extended to segmentation of the anomalous region. To be precise, the novelty of this approach lies in the fact that the GAN takes only healthy samples in the training phase, though it is capable of segmenting tumors in the “unseen” anomalous samples explaining each pixel’s contribution to the model’s prediction. Experiments have been conducted on the mini MIAS breast cancer dataset and significantly appreciable results have been obtained.
@Inbook{Mukherjee2021,
author="Mukherjee, Preeti
and Pal, Mainak
and Ghosh, Lidia
and Konar, Amit",
editor="Pedrycz, Witold
and Chen, Shyi-Ming",
title="A Generative Model Based Approach for Zero-Shot Breast Cancer Segmentation Explaining Pixels' Contribution to the Model's Prediction",
bookTitle="Interpretable Artificial Intelligence: A Perspective of Granular Computing",
year="2021",
publisher="Springer International Publishing",
address="Cham",
pages="401--425",
isbn="978-3-030-64949-4",
doi="10.1007/978-3-030-64949-4_13",
url="https://doi.org/10.1007/978-3-030-64949-4_13"
}
|
|
Multi-resolution Hierarchical Clustering by Vector Quantization
Mainak Pal*,
Preeti Mukherjee*,
Amit Konar
Advances in Cybernetics, Cognition, and Machine Learning for Communication Technologies Springer, Singapore
ICCCMLA'2019 Goa, India
project /
code /
abstract /
bibtex /
presentation
Clustering aims at grouping of objects or data-points based on a certain
measure of similarity. Existing clustering algorithms estimate the measure of
similarity of expected data-points to fall in a cluster with respect to presumed
or computed cluster centroids. Such approach of distance measure between
cluster centroid and possible data points to lie in cluster often results in
misclustering, particularly for points equidistant to multiple cluster centroids.
This paper offers an interesting solution to this problem by quantization of the
attributes of the preferred cluster centroids and then checking the existence of
the respective attributes of data-points within the quantized intervals for
possible inclusion of data-point in the cluster. This approach, referred to as
vector quantization offers additional merits of clustering at different user-
defined resolutions of data-points of varying local density. Experiments
undertaken confirm the superior performance of the proposed clustering over
the state-of-art algorithms with respect to Jaccard coefficient on breast cancer
dataset.
@Inbook{Pal2020,
author="Pal, Mainak
and Mukherjee, Preeti
and Konar, Amit",
editor="Gunjan, Vinit Kumar
and Senatore, Sabrina
and Kumar, Amit
and Gao, Xiao-Zhi
and Merugu, Suresh",
title="Multi-resolution Hierarchical Clustering by Vector Quantization",
bookTitle="Advances in Cybernetics, Cognition, and Machine Learning for Communication Technologies",
year="2020",
publisher="Springer Singapore",
address="Singapore",
pages="243--249",
isbn="978-981-15-3125-5",
doi="10.1007/978-981-15-3125-5_26",
url="https://doi.org/10.1007/978-981-15-3125-5_26"
}
|
|
Efficient Machine Learning and Neural Network Approaches for Identifying and Categorizing Offensive Language in Tweets
Preeti Mukherjee*,
Mainak Pal*,
Somnath Banerjee
Sudip Kumar Naskar
SemEval-2019 Minneapolis, Minnesota, USA
project /
code /
abstract /
bibtex
This paper describes our system submissions as part of our participation (team name: JU_ETCE_17_21) in the SemEval 2019 shared task 6: “OffensEval: Identifying and Catego- rizing Offensive Language in Social Media”. We participated in all the three sub-tasks: i) Sub-task A: offensive language identification, ii) Sub-task B: automatic categorization of of- fense types, and iii) Sub-task C: offense target identification. We employed machine learn- ing as well as deep learning approaches for the sub-tasks. We employed Convolutional Neural Network (CNN) and Recursive Neu- ral Network (RNN) Long Short-Term Memory (LSTM) with pre-trained word embeddings. We used both word2vec and Glove pre-trained word embeddings. We obtained the best F1- score using CNN based model for sub-task A, LSTM based model for sub-task B and Lo- gistic Regression based model for sub-task C. Our best submissions achieved 0.7844, 0.5459 and 0.48 F1-scores for sub-task A, sub-task B and sub-task C respectively.
@inproceedings{mukherjee-etal-2019-ju,
title = "{JU}{\_}{ETCE}{\_}17{\_}21 at {S}em{E}val-2019 Task 6: Efficient Machine Learning and Neural Network Approaches for Identifying and Categorizing Offensive Language in Tweets",
author = "Mukherjee, Preeti and
Pal, Mainak and
Banerjee, Somnath and
Naskar, Sudip Kumar",
booktitle = "Proceedings of the 13th International Workshop on Semantic Evaluation",
month = jun,
year = "2019",
address = "Minneapolis, Minnesota, USA",
publisher = "Association for Computational Linguistics",
url = "https://www.aclweb.org/anthology/S19-2118",
doi = "10.18653/v1/S19-2118",
pages = "662--667",
}
|
|
Generative Model-Driven Structure Aligning Transductive Zero-shot Learning
Omkar Gune,
Mainak Pal*,
Preeti Mukherjee*,
Biplab Banerjee,
Subhasis Chaudhuri
Arxiv, 2020
project /
preprint /
abstract /
bibtex
Zero-shot Learning (ZSL) is a transfer learning technique which aims at transferring knowledge from seen classes to unseen classes. This knowledge transfer is possible because of underlying semantic space which is common to seen and unseen classes. Most existing approaches learn a projection function using labelled seen class data which maps visual data to semantic data. In this work, we propose a shallow but effective neural network-based model for learning such a projection function which aligns the visual and semantic data in the latent space while simultaneously making the latent space embeddings discriminative. As the above projection function is learned using the seen class data, the so-called projection domain shift exists. We propose a transductive approach to reduce the effect of domain shift, where we utilize unlabeled visual data from unseen classes to generate corresponding semantic features for unseen class visual samples. While these semantic features are initially generated using a conditional variational auto-encoder, they are used along with the seen class data to improve the projection function. We experiment on both inductive and transductive setting of ZSL and generalized ZSL and show superior performance on standard benchmark datasets AWA1, AWA2, CUB, SUN, FLO, and APY. We also show the efficacy of our model in the case of extremely less labelled data regime on different datasets in the context of ZSL.
@misc{gune2020generative,
title={Generative Model-driven Structure Aligning Discriminative Embeddings for Transductive Zero-shot Learning},
author={Omkar Gune and Mainak Pal and Preeti Mukherjee and Biplab Banerjee and Subhasis Chaudhuri},
year={2020},
eprint={2005.04492},
archivePrefix={arXiv},
primaryClass={cs.CV}
}
|
|
Operator Learning for Robust Reinforcement Learning
Dec'23 - Present
Trained a neural operator to solve the Hamilton-Jacobi-Bellman (HJB) equation, where the solution effectively maps from the state functional space to the action functional space. By experimenting on a perturbed system, we analyzed the performance of the adapted policy, demonstrating the robustness and adaptability of our approach. Furthermore, we are extending this methodology to simultaneously solve the HJB and Fokker-Planck (FP) equations within a coupled system, aiming to provide a comprehensive solution that addresses both the optimization and the probabilistic dynamics of the system.
Supervisor: Dr. Vijay Gupta
|
|
Procative Team Support in Human Robot Teaming
Aug'22 - Present
Designed a Bayesian Model for intention prediction in Human-Robot teams, focusing on developing policies for the assistive robot based on inferred intentions. Currently working on extending the model to work seamlessly in team settings.
Supervisor: Dr. Vijay Gupta
|
|
Resilient Multi-agent Reinforcement Learning with Function Approximation
Aug'23 - Jan'24
Worked on the development of a resilient consensus-based actor-critic algorithm, specifically designed to mitigate the adverse effects of Byzantine agents within cooperative networks in the context of multi-agent reinforcement learning. This involved enhancing the simulation framework to facilitate comparisons with the current state-of-the-art methodologies, thereby ensuring a comprehensive evaluation of the algorithm's efficacy in maintaining network integrity and performance under adversarial conditions.
Supervisor: Dr. Vijay Gupta
|
|
Data augmentation using GAN to improve Generalizability and Robustness of Finger-Induced Motor Imagery Classification
Aug'20 - May'21
Developed a generative network to generate synthetic fNIRS data that are very similar to original data. Designed an end-to-end paradigm to perform classification based on the image biomarkers for finger tapping tasks.
Bachelor's thesis Supervisor: Dr. Amit Konar
|
|
Automatic behavioral analysis of C.Elegans locomotion
May'20 - Sep'20
Worked on various recurrent neural models and autoregressive models to automate behavioral analysis of C.Elegans locomotion.
Supervisor: Dr. Thomas Serre
|
|
Generative adversarial approach for unsupervised domain adaptation
June'20 - Dec'20
Extending our previous work on ZSL in unsupervised domain adaptation. Working on various generative models to achieve better latent layer representation of multimodal visual feature space.
Supervisor: Dr. Biplab Banerjee
|
|
Computational Vision in Cryo-electron Tomography
Jan'20 - Dec'20
Exploring various methods to extract information from tomographic data.
Supervisor: Dr. Min Xu
|
|
Zero-shot breast cancer segmentation
Nov'19 - Mar'20
Trained the BiGan model on healthy data so that the trained model can construct nearest healthy samples from unhealthy data. Based on RISE model, we proposed a novel architecture for automatic segmentation of the tumor region from our previous understandings. Our approach is capable of segmenting tumors without using any unhealthy samples while training.
[publication]
Supervisor: Dr. Amit Konar
|
|
Trunsductive Zero-shot learning
May'19 - July'19
Explored zero-shot learning application on various domains. Implemented various autoencoders on latent space and semantic space. Extended the Structure Aligning Discriminative Latent Embedding for Zero-Shot Learning in transductive settings.
[publication]
Supervisor: Dr. Biplab Banerjee
|
|
Vector Quantization Clustering
Mar'19 - Feb'20
In existing clustering algorithms, larger attributes have more contribution in the distance measure in comparison to the attributes of small values. Thus, attributes of smaller values even if differ by larger magnitude are not encountered in the clustering algorithms - causing false clustering. To overcome this problem, we have proposed an novel clustering algorithm based on quantization at each attribte level. Our approach performs better than state-of-arts and also computationally less expensive. We have worked on several gene-micro array datasets, breast cancer dataset. Proposed method is also helpful in time-series modeling.
[publication]
[manuscript in preparation]
Supervisor: Dr. Amit Konar
|
|
Identifying and Categorizing Offensive Language in Social Media
Sep'18 - Feb'19
Worked on sentiment analysis of tweets. Explored multiprocessing. Explored different techniques of machine learning ( like Logistic Regression, Linear SVC, LinearSVC with L1-based feature selection, Multinomial NB, Bernoulli NB etc. ). Implemented several Deep Learning networks like CNN-word2vec, attention based Bi - RNN with LSTM. Our team participated at task 6 on SemEval-2019. Tasks were three fold. First, Offensive language identification. Second, if the tweet is offensive, then automatic categorization of whether that is targeted or not. Third, if the tweet is targeted, then target of offence identification, like: is it targeting individual, group or others? Our methods performed well and task description paper published at SemEval.
[publication]
Supervisor: Dr. Sudip Kumar Naskar
|
|
Some exploratory voyages...
Perro Gato : An Image Classifier [code]
Real-time 2D plot of Azimuth Plane using Ultrasonic Wave Sensor (HC SR04) [code] [demo]
Approximate-Pi [code] [demo]
Cloud : A nodejs app deployed aiming to help beginners finding Open Source projects [code] [demo]
Mosom : A weather forecast app built in PyQt [code]
Shoot The Ball : A game developed using Processing [code] [demo]
|
|